Delivering digital solutions to Australia’s greatest health challenges.
We’re recognised by health systems in Australia and across the
globe as leaders in digital health research, tool and platform
development and implementation.
We have world-leading capability in areas such as:
- clinical terminology and data interoperability
- health data analytics
- clinical image analysis
- genomic data analysis and engineering
- biostatistics
- mobile health and tele-health
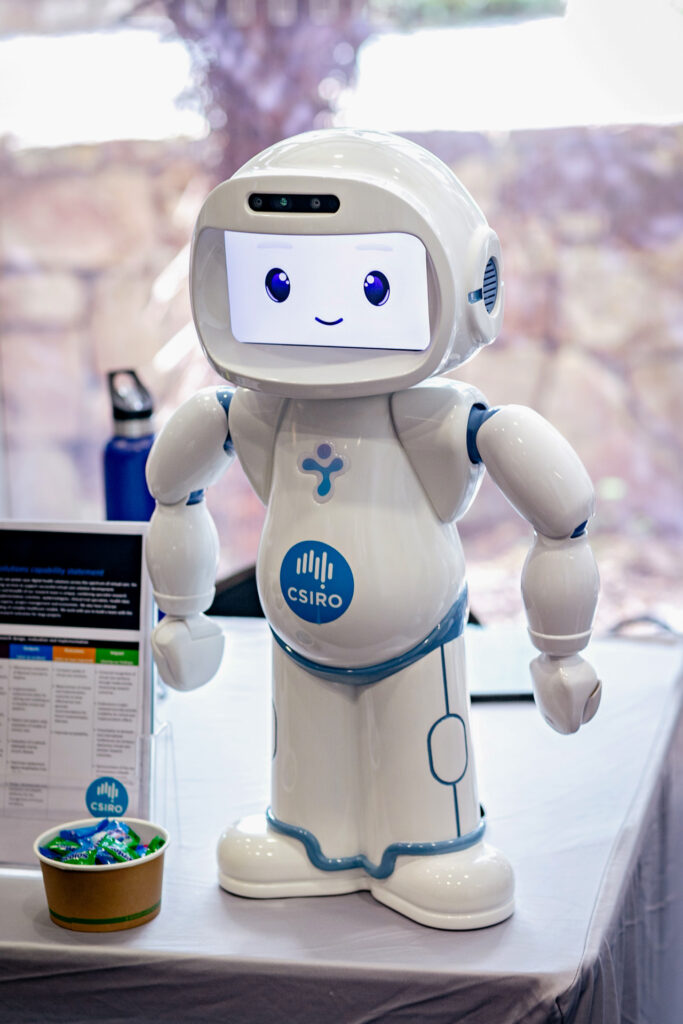
The Australian e-Health Research Centre (AEHRC) is CSIRO's digital health research program and a joint venture between CSIRO and the Queensland Government. The AEHRC works with state and federal health agencies, clinical research groups and health businesses around Australia.